Overview
The fleet management industry was rapidly evolving, with OEMs tightening control over vehicle data, AI reshaping operations, and fleets demanding smarter, higher-ROI solutions. As competition intensified and base services became commoditized, Zonar’s legacy SaaS offering and new platform solution faced new constraints.
To stay ahead, Zonar needed to offer flexible data solutions, predictive analytics, and task automation. To better understand what this would look like, Zonar explored an AI-first strategy to create an AI-assisted decision-making layer across data gathered from its telematics units, tablets, and smart cameras.
To explore AI's role in Zonar’s innovation, Amanda Parkhurst and I led a multi-stage workshop to generate AI solutions for customer value.
Business Value: Product Vision
Team: Myself & Amanda Parkhurst; participants
Contributions: UX Design, Visual Design
“What would it look like if AI was a core part of our platform’s offering?”
An A.I. First Approach
AI First Product Thinking
To take a true AI first approach to rethinking our product experience, we needed to consider the overlap between the domain knowledge and associated data, the Job To Be Done, what AI capabilities provide to improve those jobs, and how we might approach the user experience to support the new capabilities with technology patterns that users might be less familiar with.
Questions we needed to ask:
What does our product look like if AI was the core offering instead of an add on?
What would the experience look like if it continuously learned and adapted to users needs?
What would it look like if the experience proactively predicted users needs?
How does the human fit in the “loop”?
What are the ethical considerations of this kind of approach?
Work Session
Identifying pain points and business value
We began the session by encouraging participants to explore various AI applications and consider how they could address challenges faced by Zonar’s customers.
“The focus of the framework is not to start with the technology and try to force-fit it into a solution. Rather, it starts with the problem and works its way forward”
We then shifted the focus away from the tech-first warm up to dive deeper on customer’s problems, irrespective of technology.
When AI is probably better (from Google)
Recommending different content to different users.
Prediction of future events.
Personalization improves the user experience.
Natural language understanding.
Recognition of an entire class of entities
Detection of low occurrence events that change over time.
An agent or bot experience for a particular domain.
Showing dynamic content is more efficient than a predictable interface.
Adding Structure
Critical questions to add structure
After narrowing down key use cases from the pain point exercise, we needed to explore different ways to present AI UX patterns. We also needed to consire how these patterns align with the Job To Be Done and the temporal modes of data required to complete the task.
High Fidelity AI “Copilot” Comps
The questions above led us to rethink the desktop experience that is comped below. The focus for agentic AI follows both HAX Copilot concepts as well alerting through social media channels like we see in Tableau Pulse.
Home Page Dashboard with embedded metrics and Assistive AI
Use Case: Triage home page with “Z-Pilot” button on upper right hand side to access assistant.
Home Page (Cont.) with embedded metrics with assistive AI open
Mobile alert with metrics analysis and AI agent for further exploration
Use Case: User gets alerted to trend in driver behavior via mobile. They could use assistive AI to further analyze the situation….
…or go to desktop Posted Speed report with embedded AI (analysis highlights in modules) and “Z-Pilot” button with tabular data to explore the trend analysis more deeply….
…and then could go to immersive AI “Z-Pilot” experience in the historical tabular data view to further interrogate the data.
Query results with AI guidance, tabular data pushed down but still accessible
Agentic Workflow Concepts
Automation and agents AI idea in the field
In addition to the desktop scenario comped above, we also generated ideas and story boards for practical problems our customers face based on data we can gather today. We aligned those concepts with the archetypes below.
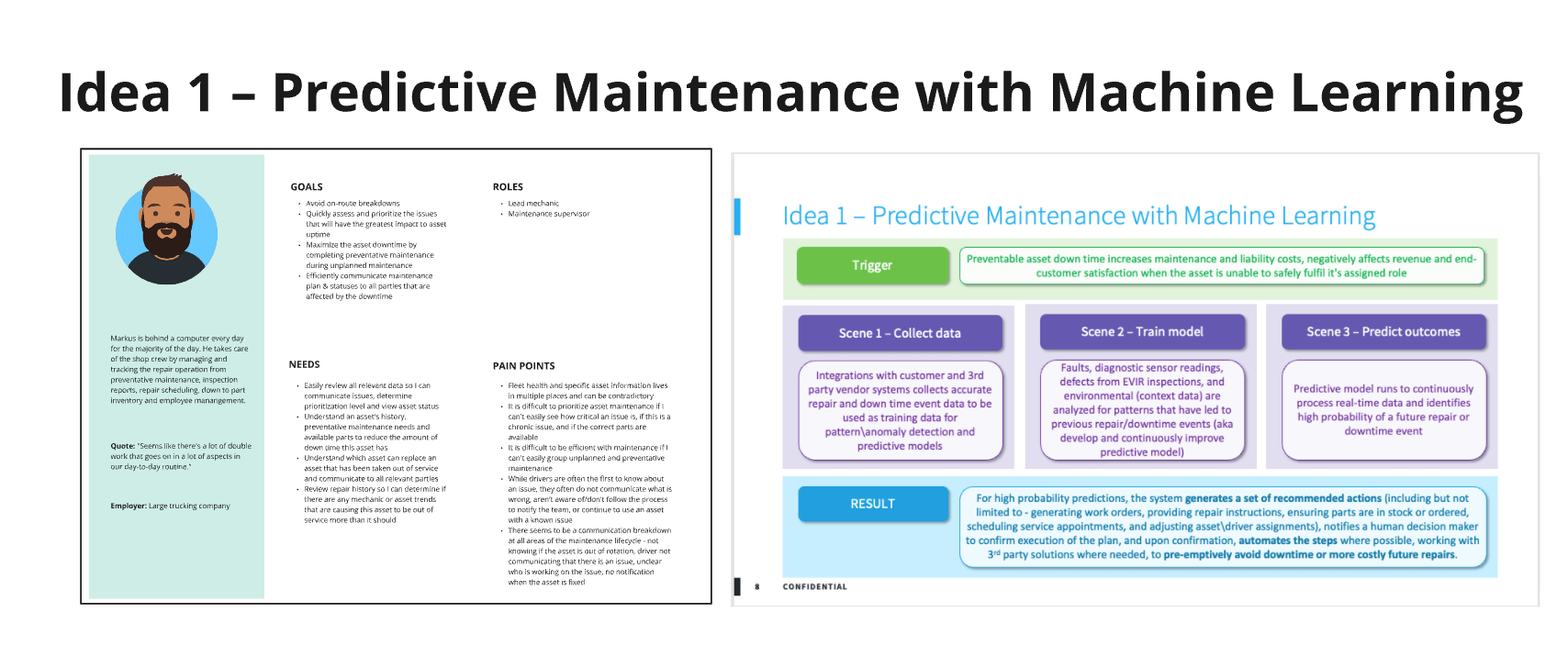


Envisioning the user experience
We tested these ideas with customers at our annual Zonar Together Conference with users to better understand their attitude towards AI and Zonar products. To help customers better understand and assess the concepts, I envisioned how the concepts would work and translated them into story boards that were posted at a display at the conference.
Results
The customer conference had professionals from diverse roles—transportation coordinators, fleet managers, IT specialists, mechanics, maintenance planners, and engineers.
The first day set the stage with discussions on AI familiarity, perceptions, and whether companies were actively pursuing AI-driven solutions. While some saw promise in areas like predictive maintenance, data integrity, and automation, others remained cautious about its implementation.
On the second day, following an AI presentation by Zonar to customers, reactions were mixed—some found it insightful, while others expressed uncertainty about AI’s capabilities. Conversations then shifted to Zonar’s potential AI initiatives, with attendees showing varying degrees of interest in leveraging AI for predictive maintenance, data analysis, and operational enhancements.
Next Steps
This new idea represents an AI First approach to meeting the customers needs, but we would need to perform various exercises to determine feasibility and viability. The agent workflows need to be further explored as they had a better defined scope and understood set of challenges to evaluate.
Questions
Agentic AI probably isn’t chat interfaces for the more complex fleet management workflows…how can we support those?
How does the experience change for different roles?
How can we ensure it is safe and ethical?